Avoiding AI Integration Failure: Strategies for Success
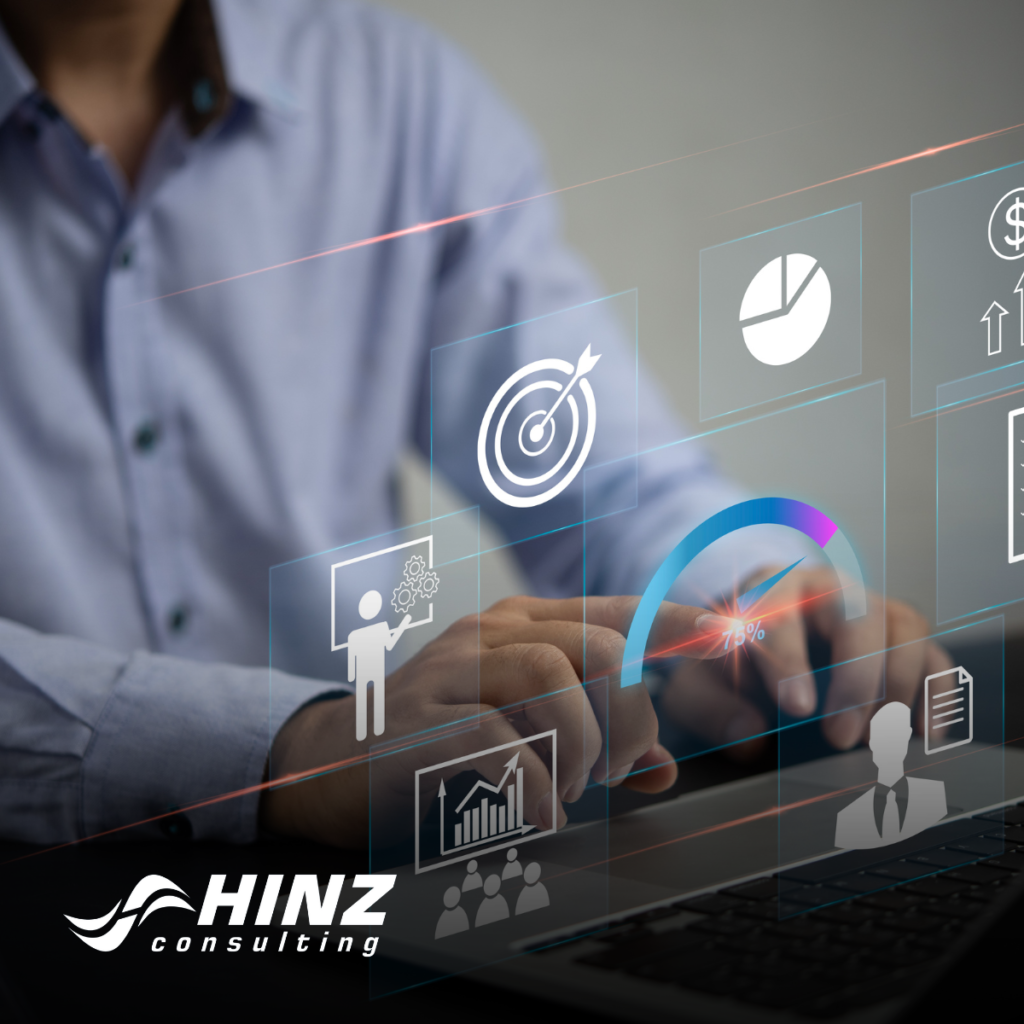
In the ever-evolving landscape of technology, the integration of Artificial Intelligence (AI) has become a strategic imperative for organizations seeking to stay competitive and innovative. However, the journey towards AI integration is not without its challenges. In this blog, we will explore key strategies to help your organization successfully navigate the complexities of AI adoption, […]
Simple Steps for Platform Security in Line with NIST Standards
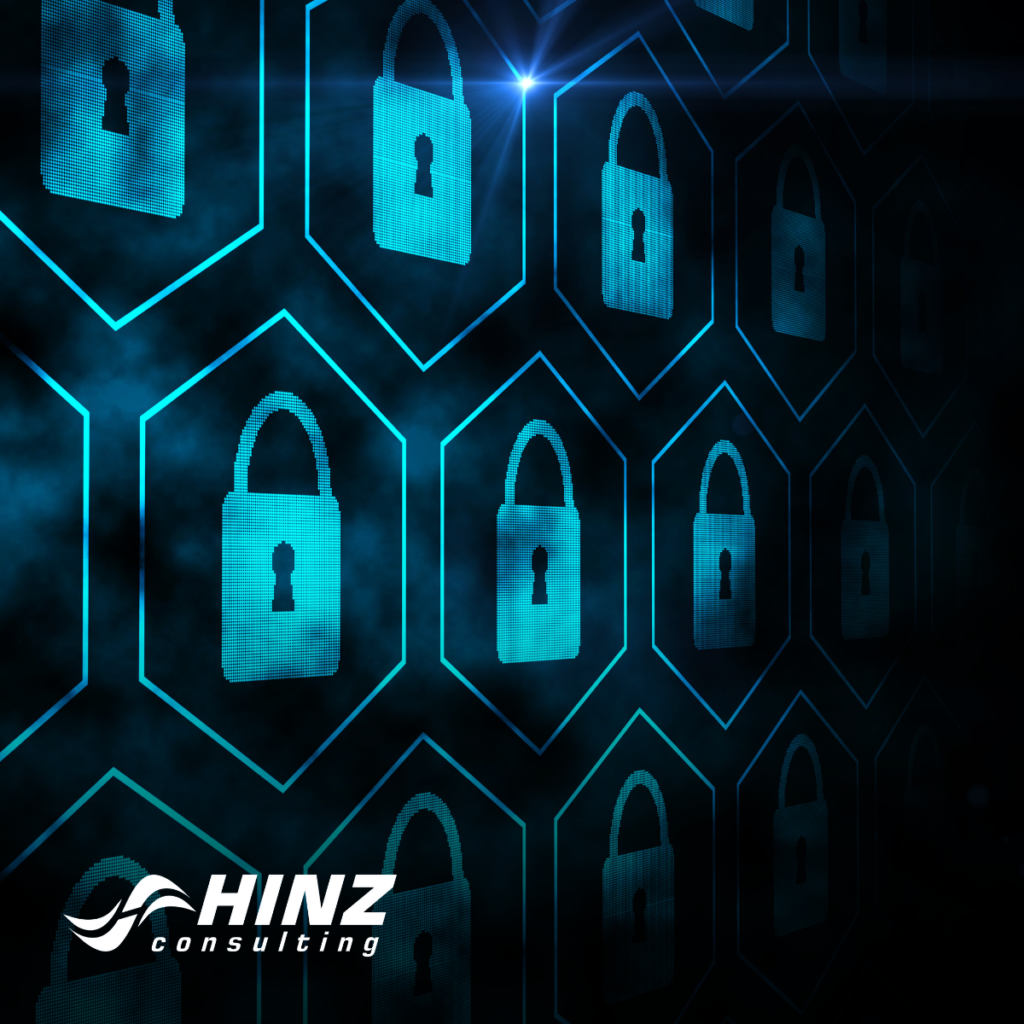
In the world of government contracting, where technology and sensitive information intertwine, keeping our digital platforms safe is more important than ever. Let’s break down the key aspects of Platform Security and how following straightforward guidelines, like those laid out by NIST, can make a significant difference. Platform Security Made Simple for Government Contractors Navigating […]
Navigating the Risks: What Happens When Your Proposal AI Talks to the Internet?
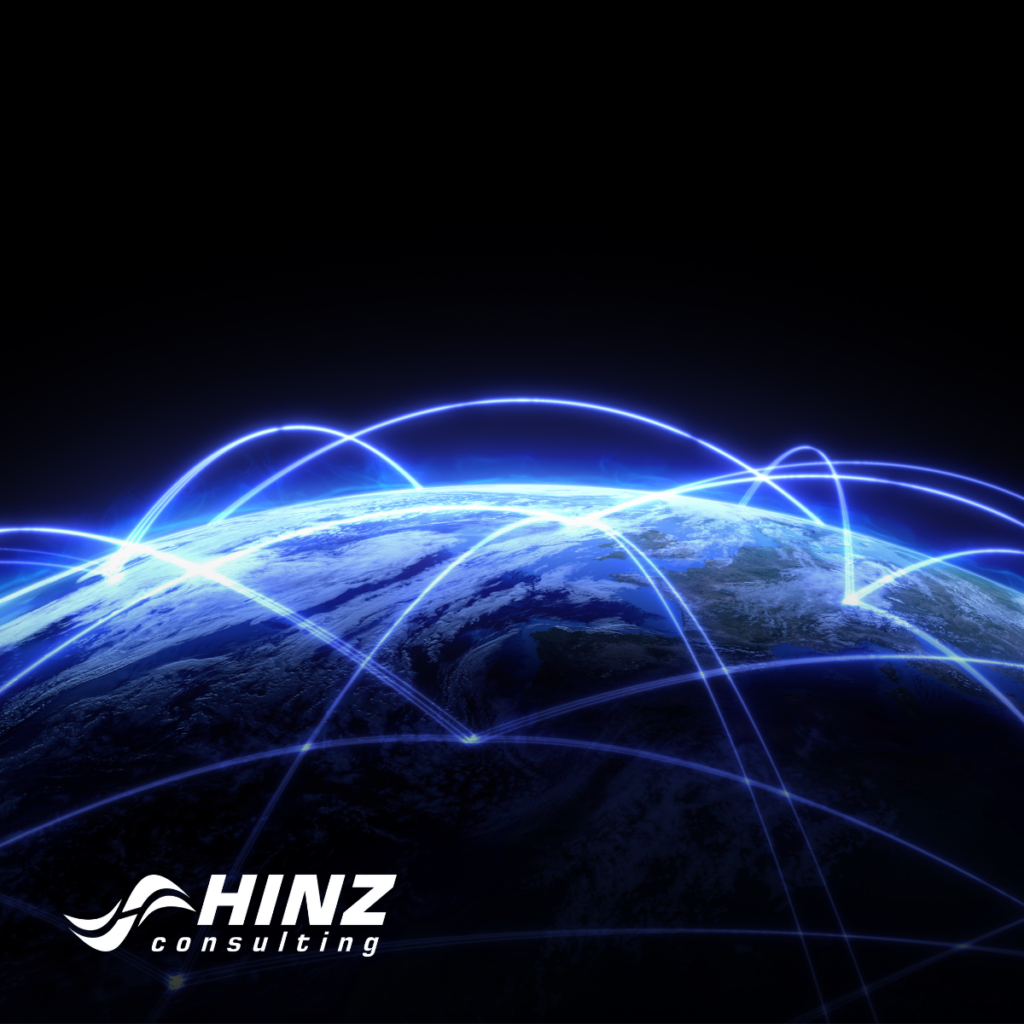
If an AI interacts with the internet to gather information for proposal generation, it might not always tailor its solutions specifically to your organization’s offerings. Here’s how this factor can influence the dynamics: Generic Solutions: Lack of Context: Customization Challenges: Dependency on Training Data: Human Oversight: Customization Features: Integration with Internal Data: Balancing the benefits […]
Maximizing Perceived Customer Value vs. Price
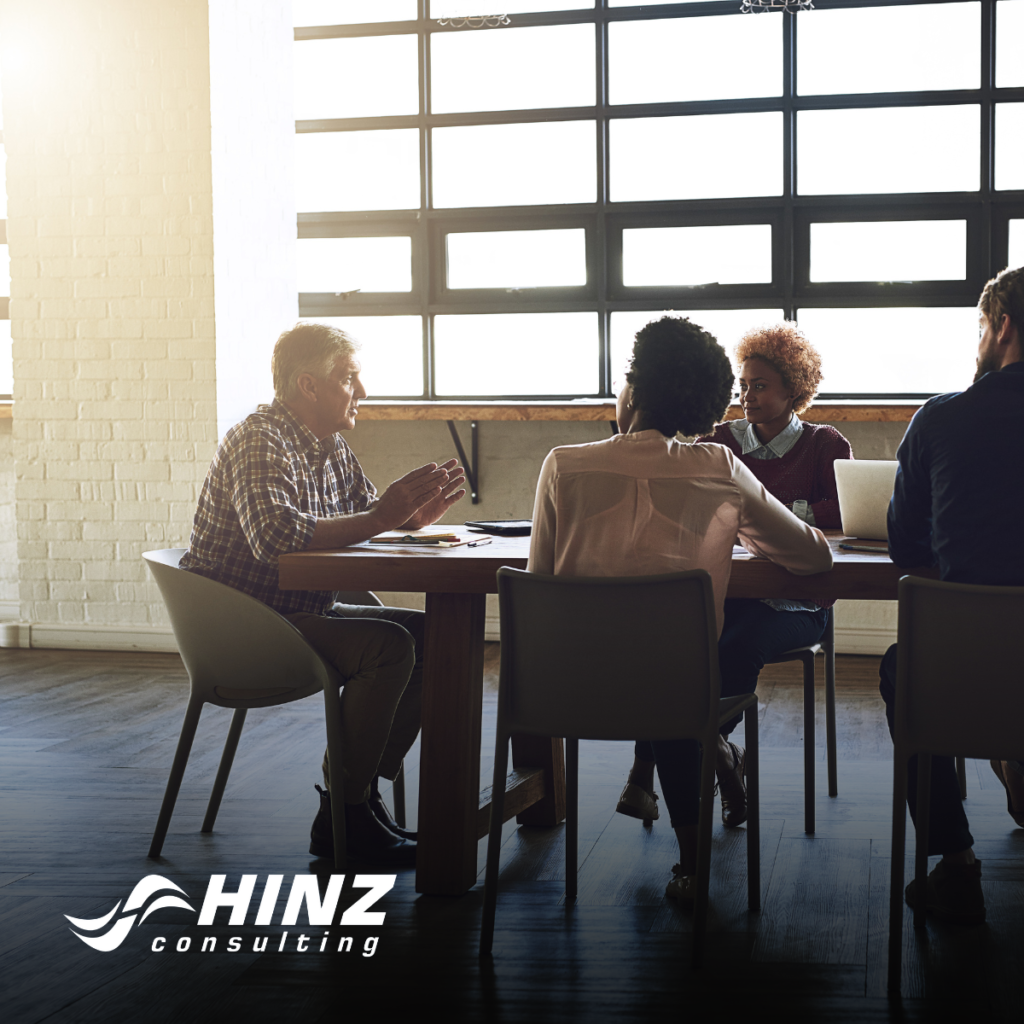
I recently had the opportunity to work with the team overhauling the Association of Proposal Management Professionals (APMP) Competitive Price to Win Book of Knowledge. We spent a significant amount of time discussing value versus price, particularly when a customer is conducting a competitive procurement. We all agreed that maximizing the difference between the value […]
What Is The Hidden Markov Model?
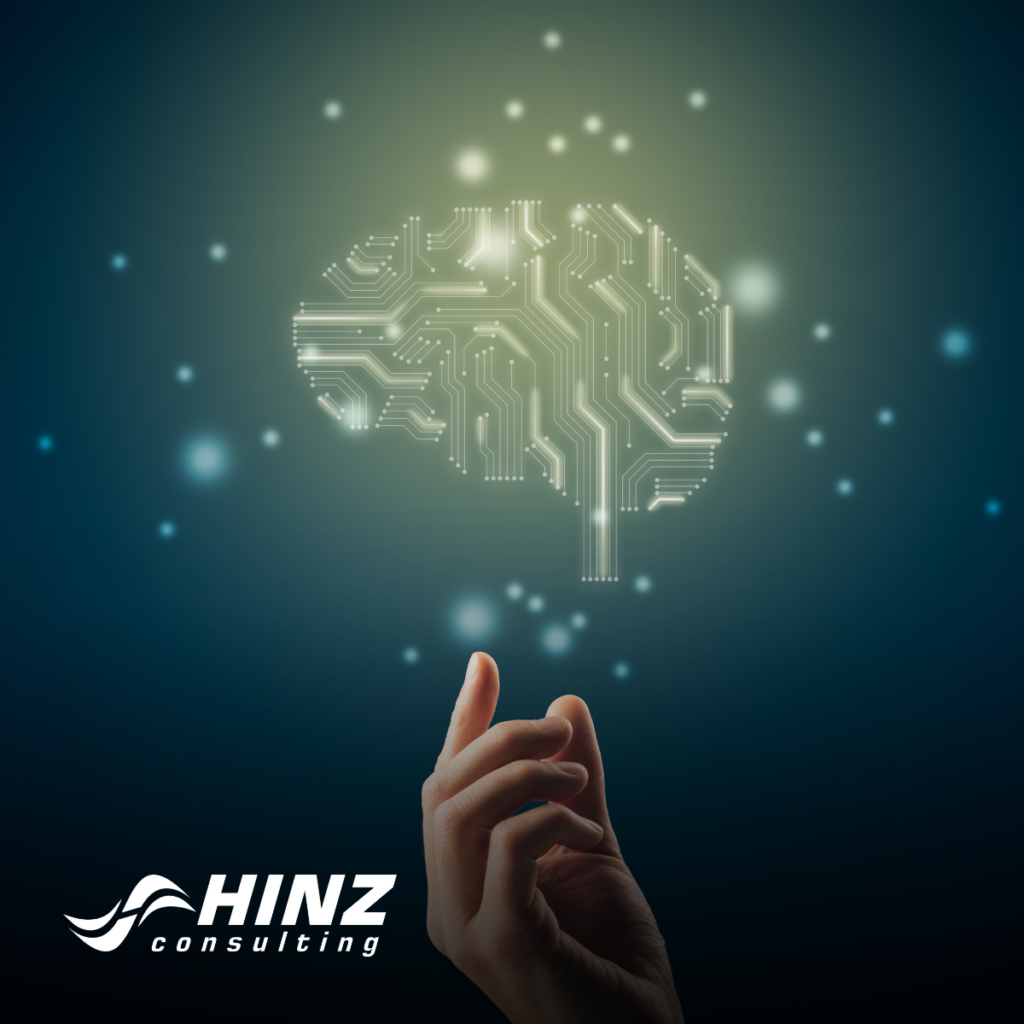
Hidden Markov Models (HMMs) are a fascinating concept in the realm of artificial intelligence, renowned for their versatility and applicability. Let us uncover the key aspects of HMMs: Understanding the Basics · Definition: HMMs are statistical models that represent systems with hidden states, observable outputs, and transitions between states. They operate under the assumption of […]
What Are The Pitfalls Of Using AI?
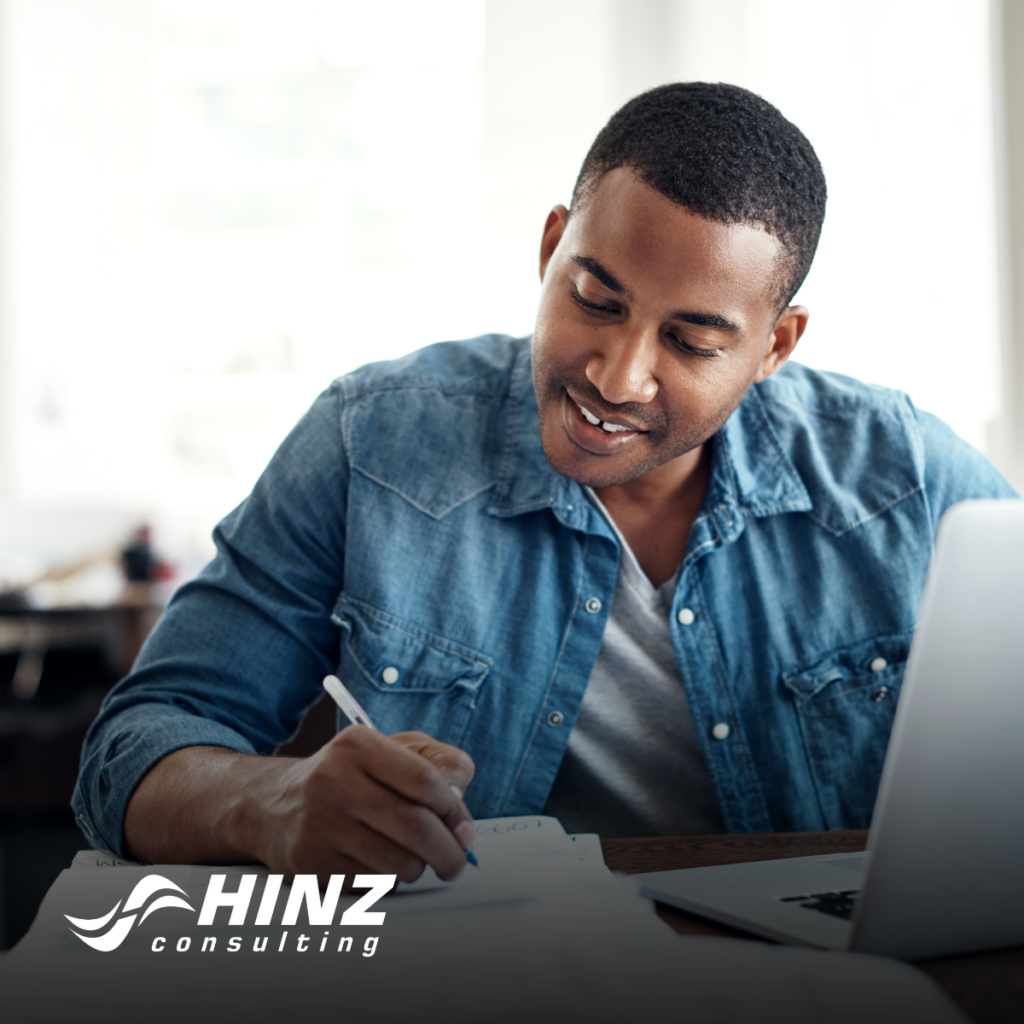
Implementing AI in business processes can be transformative, but it is essential to be aware of potential pitfalls. Let us explore common challenges associated with AI and strategies to mitigate them: 1. Data Quality and Bias: · Challenge: Poor data quality or biased datasets can lead to inaccurate AI predictions, reinforcing existing biases. · Mitigation: […]
Understanding Fuzzy Logic in AI:
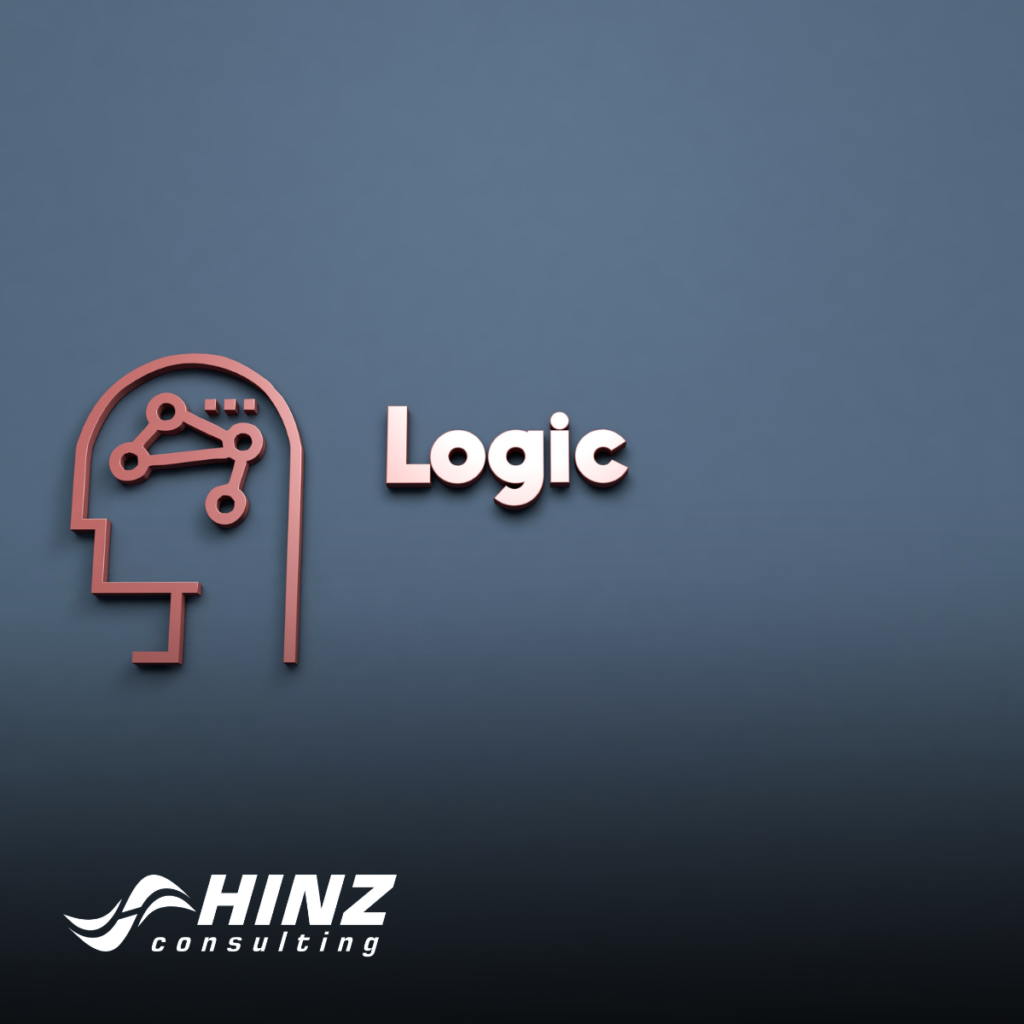
Definition · Nuanced Decision-Making: Fuzzy logic is an approach that deals with uncertainty and imprecision, allowing systems to handle shades of truth between true and false. · Degrees of Membership: Concepts are not just true or false but can have varying degrees of membership in a set. Core Elements · Membership Functions: Assigning degrees of […]
The Symbiosis of AI and Learning
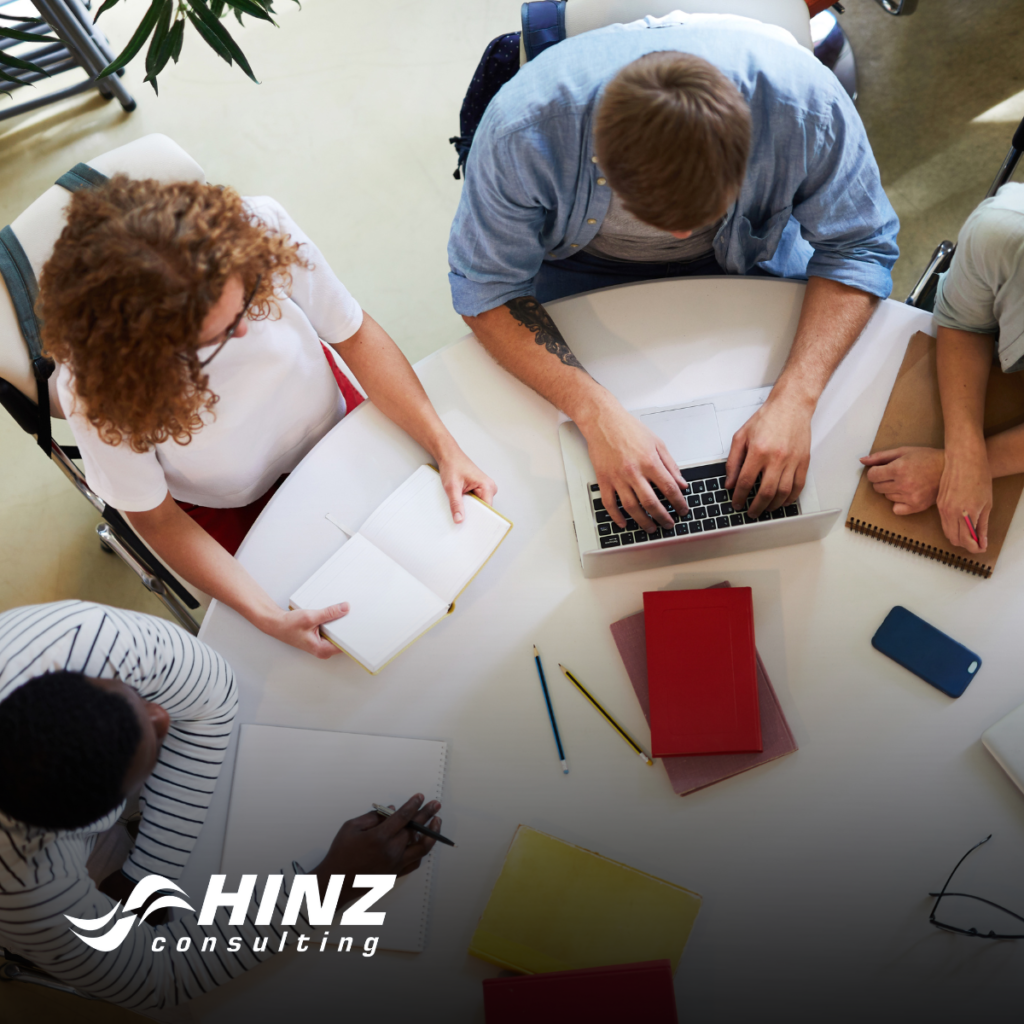
Embark on a journey into the transformative synergy between artificial intelligence (AI) and the process of learning. In this quick reference document, we touch on how AI augments and reshapes learning paradigms across various domains. AI’s Pivotal Role in the Learning Landscape In the ever-evolving landscape of education and beyond, AI has emerged as a […]
AI’s Evolution in Representation and Reasoning
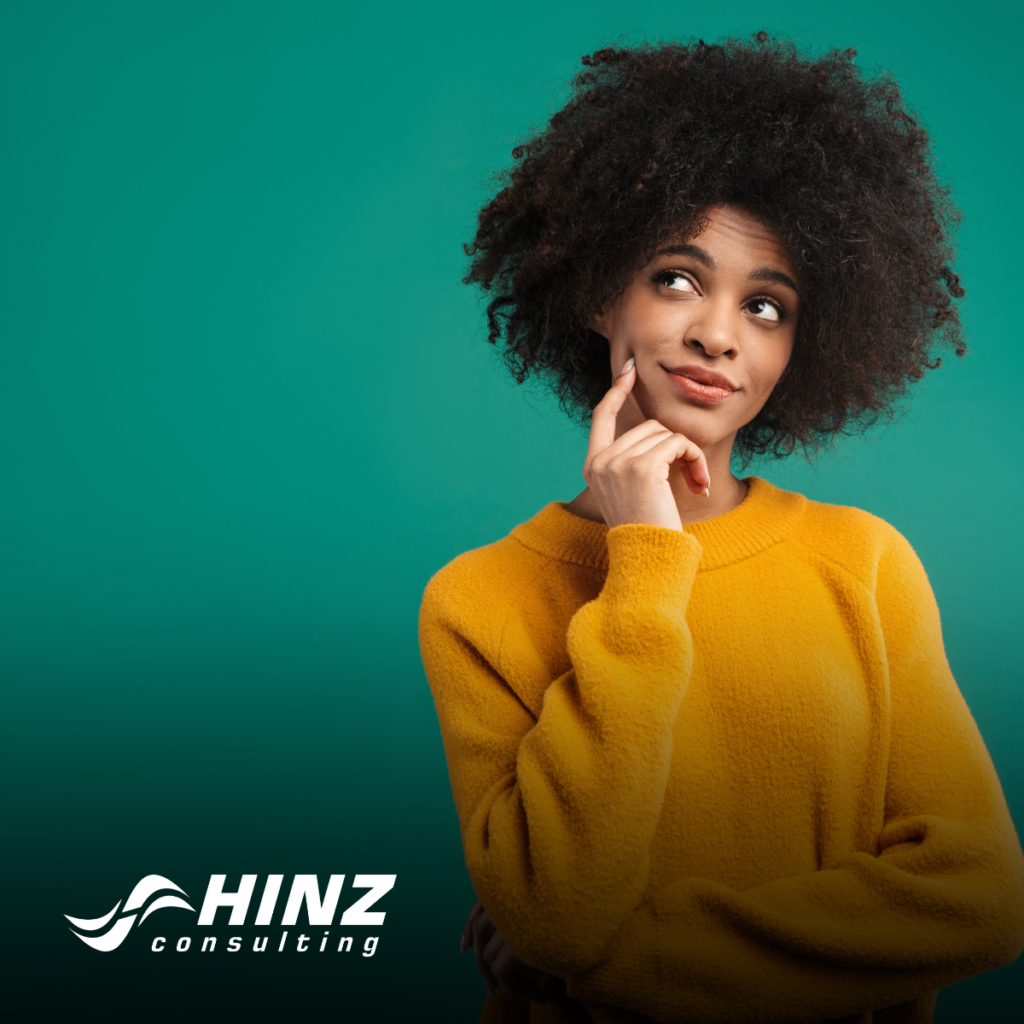
In the realm of artificial intelligence (AI), the dynamic interplay between representation and reasoning forms the backbone of intelligent systems. Let’s embark on a journey into the intricate landscape where AI processes information, understands context, and makes decisions. The Essence of Representation and Reasoning in AI Representation involves translating the world into a format AI […]
The Intricate Dance of AI and Perception
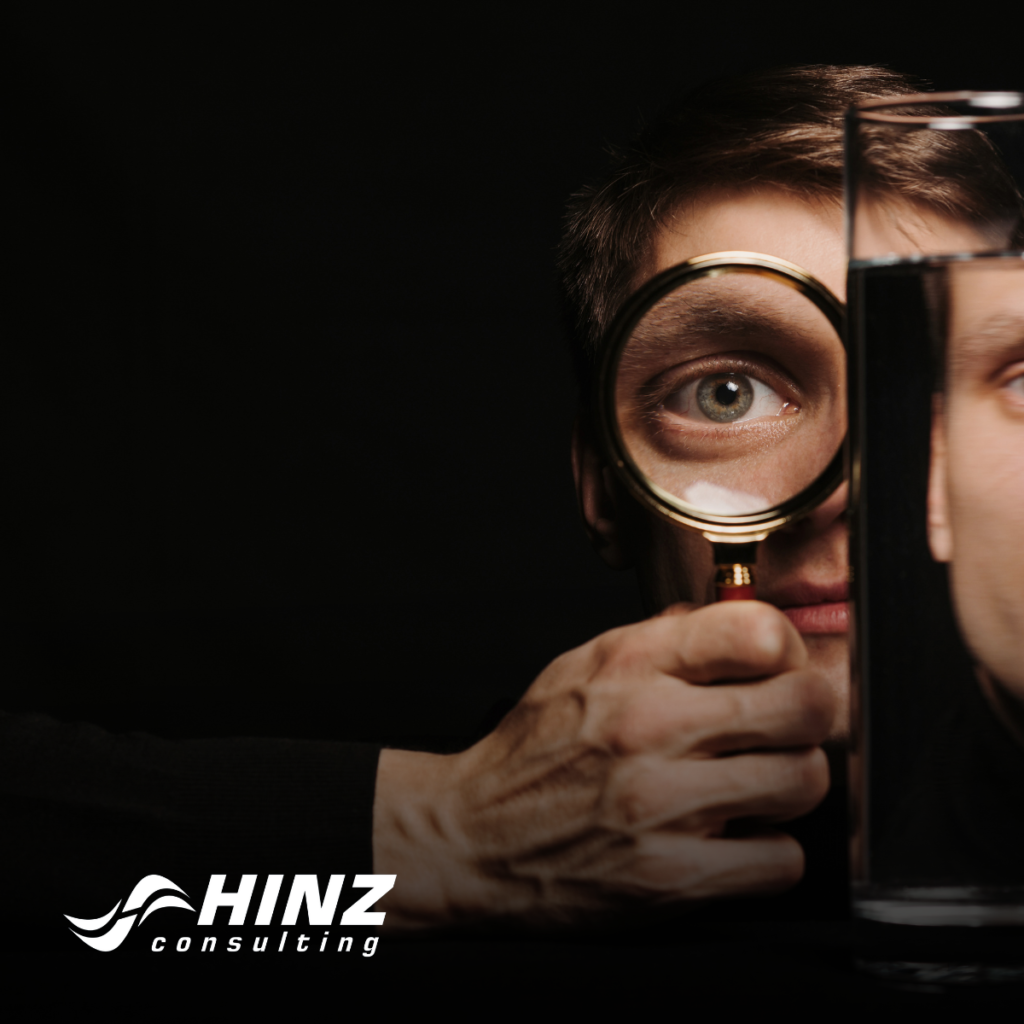
The concept of perception in AI is a central hallmark, reflecting how machines interact with and interpret the world around them – the human world. Let’s delve into the nuanced relationship between AI and perception, exploring the mechanisms that enable machines to make sense of their environment. Deciphering Perception in AI Perception in AI mirrors […]